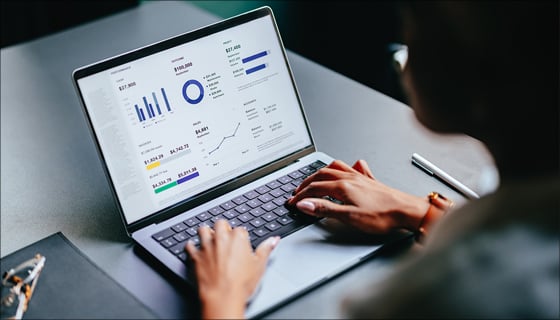
In Part One of our blog series, we explored why insurance pricing is hard — not because insurers lack sophisticated models, but because of disconnects in how those models are implemented and maintained. In Part Two, we looked at the confusion caused by inconsistent terminology and the need for clearer conversations across teams. Now, in Part Three, we bring it all together with a framework for evaluating and strengthening your pricing function — helping you identify what's getting in the way, and what to do about it.
If you're not completely happy with your current pricing process, you're far from alone. Maybe pricing changes take too long to implement. Maybe you’re not confident in the accuracy of your premiums. Or perhaps the market is evolving faster than your pricing can keep up. Each of these symptoms points to a different part of the process — and solving them starts with figuring out where the real problem lies.
Is Speed or Accuracy the Bigger Challenge?
For most insurers, pricing challenges fall into two categories: pace and accuracy.
Speed, or pace, refers to how quickly rates can be reviewed and implemented. In fast-moving markets, reducing the lag between identifying optimal rates and getting them into production can be a serious competitive advantage. For some insurers, this is the biggest issue. They trust their models — but struggle to get new rates out the door in a timely manner, before they’re outdated.
Others have concerns about the accuracy of the rates themselves. They may lack confidence in whether their premiums are competitive, or sustainable. Rising loss ratios and a weak market position are often the first clues that something isn’t working.
Of course, pace and accuracy are often connected. Old rates tend to be inaccurate by definition. But thinking about them as separate problems can help you pinpoint the root cause.
Getting Analytics to Move at Market Speed
Some organizations struggle because it takes them too much time to produce a predictive pricing model. Others have the opposite problem — they create plenty of models, but can’t get them into production fast enough to make a difference. In the first case, the issue lies within analytics. In the second, it’s an execution problem.
Long analytics timelines are often the result of poor data access or outdated tools. Most insurance data systems aren’t structured for easy analysis, especially when data is stored across multiple platforms or inherited through acquisitions. Actuarial teams may spend weeks just assembling datasets before they can begin actual modeling.
Supporting analytics starts with giving them the tools — and the time — they need to do their jobs well. That means investing in data extraction capabilities, reducing dependence on IT, and creating multi-disciplinary teams that combine technical and business expertise.
Even when data access isn’t a problem, analytics projects can still be slow. Generalized linear models (GLMs), while powerful, require a lot of iteration and hands-on work. Add in documentation, governance, and peer review — all essential for trust and transparency — timelines stretch further. Open-source tools like Python and R are flexible but don’t help with collaboration and workflow management.
That’s why leading insurers are turning to platforms built specifically for pricing — platforms that streamline automation, foster collaboration, and embed governance from the start.
When Execution Breaks Down
But what if your analytics are strong, and your models are solid — yet pricing changes still take forever to go live? Then you're dealing with an execution bottleneck.
In personal lines, this usually shows up during the decision-making phase. After analytics teams generate a new set of rates, business leaders must review and approve them. Without a structured, transparent process, this phase can turn into a bottleneck. Delays in feedback, second-guessing, or lack of trust in the analysis can all hold things up.
Open, frequent conversations between business and analytics are key. So are platforms that enable quick, iterative review cycles — and a clear definition of when a rate is “ready enough” to move forward. When teams are aligned on goals and expectations, decisions happen faster and with greater confidence.
In commercial lines, the bottleneck is more often tied to implementation. Underwriters play a central role in pricing, and many still rely on spreadsheets or highly customized tools that vary by team or market. That makes the pricing process harder to standardize — and harder to scale.
Here, the most effective improvements involve consolidating workflows. Centralized underwriting workbenches, connected pricing platforms, and AI tools that streamline submission prioritization can reduce friction without sacrificing underwriting judgment. The goal isn’t to replace expertise — it’s to support it with better tools.
The Hidden Hurdle: Rate Deployment
Even after pricing decisions are made, the final handoff to production can be surprisingly difficult. Most rating engines weren’t designed for business user flexibility. They prioritize speed for quoting — but not for easy updates. As a result, even small changes can become full-blown IT projects.
Worse, analytics tools like R and Python, while excellent for modeling, aren’t built to integrate with rating engines or policy admin systems. So the same rates that were approved may still take weeks or months to actually hit the market.
To overcome this, insurers need rating plans that are easy to update, structured for automation, and flexible enough to support both personal and commercial lines. Ask yourself: how easily can your team make changes? Who owns rate updates — the business or IT? Can your systems keep pace with the market?
The answer may reveal more than a process issue — it could expose a strategic risk.
What About Pricing Accuracy?
While speed gets most of the attention, accuracy problems can be just as damaging. Maybe you’re not sure whether your prices are too high to compete or too low to be sustainable. Maybe your loss ratios are creeping up, but you don’t know why.
Sometimes, the problem is a lack of confidence — not in the business, but in the visibility and clarity of the process and the data. Executives might hesitate to green-light pricing changes if they don’t fully understand the analysis. That’s why business leaders should feel comfortable asking questions like: How do we know this rate is right? And analytics teams should be prepared to answer those questions clearly and directly.
When business and analytics teams are aligned, those conversations build trust — and lead to better decisions. But if your company’s performance is deteriorating, the problem could be deeper.
Addressing the Real Risks: Mispricing and Adverse Selection
Mispricing doesn’t always mean your average rate is too low. It might mean you’re not segmenting well — charging the wrong price to the wrong customer. That opens the door for competitors to cherry-pick your best risks, leaving you with a more volatile, higher-risk book. This is adverse selection in action.
Insurers that fail to keep pace — or fail to price accurately within segments — often see loss ratios rise, even when overall rates seem reasonable. That’s why rate adequacy and segmentation both matter. The right tools, strong models, and a responsive process are your best defenses.
Pricing That Performs and Evolves
As we’ve seen across this three-part series, insurance pricing is complex not because the math is hard, but because the process is fractured. It spans teams, systems, and timelines — daily, for quoting and binding, and periodically, for rate reviews and updates.
In Part One, we explored why this complexity of pricing creates friction. In Part Two, we argued that clearer language and shared understanding are essential to excellence in pricing. Here we’ve laid out a framework to evaluate what’s really slowing you down.
The takeaway? Every pricing issue is fixable — with the right insight, the right questions, and the right tools.
Because when your pricing function is aligned, agile, and accountable, you gain more than efficiency. You gain confidence — to act faster, compete smarter, and price with precision.