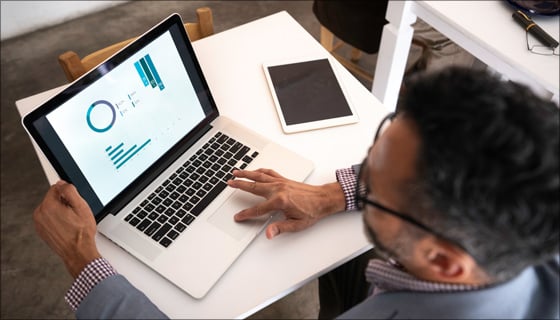
Most insurers are invested in developing risk models but despite their achievements, many are failing to progress their models and expand their application over time. As a result, they could be losing out on substantial value creation.
At the core of the issue is the fact that most data scientists/analytics experts thrive in creating innovative risk models but are not always as skilled at the more mundane work of implementing and monitoring those models. Carefully crafted risk models create little business value if they never get implemented or end up being one-time efforts that grow stale over time. When a risk model is created to solve a business problem, it should always be viewed as an ongoing program and effort.
Insurance executives should be asking: How are we managing our risk modeling program? And can we be doing things more efficiently and effectively?
We recommend, as a foundation, a next-generation risk modeling program should continuously consider:
The Problem – When you address a new business problem, expertise and resources are necessary to come up with an intelligent solution. Using data scientists and analytics experts, although costly, is appropriate for scoping the problem, gathering data, and creating a predictive model via predictive analytics.
Implementing Analytics – Implementing predictive models has become easier over time and there are now standard approaches insurers can leverage, but the application and maintenance of such risk models should not be an afterthought. Know how the predictive model will be implemented before you begin and consider how best to supply the needed data and integrate the results with your core systems and business.
Monitor Performance – It is imprudent to assume a predictive model will work exactly as expected in the design phase. Surprises and unintended consequences are the norms in all business undertakings. Well-performing models may drift or change over time, particularly as your business evolves. Expect changes and find technologies and resources to support the upkeep of your models and program.
Refresh the Model – Monitor your progress, and when the monitoring indicates, refresh your data and predictive model. Even if comprehensive re-working is not required, revisions likely will be needed. Insurers need to expect and plan for refreshes of predictive models whenever they tackle a business problem.
Reconsidering the Business Problem – Eventually, it may be decided that the approach taken to build the original predictive model could be substantially improved if reconsidering the problem from scratch. Here the data scientists and analytics experts can be brought back in to exercise their specific skills.
Over time, multiple predictive models will be required to address a business problem. And centering on the business problem, not just the model, is the right approach.
Taking burdens off your data scientists and analytics experts will free them up to address other problems and opportunities in your business. Applied thoughtfully, predictive analytics and risk models can continue to transform your business in new ways and new areas.