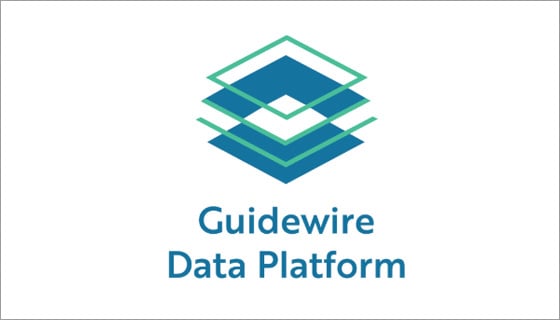
We live in a data-driven world and enterprises are realizing that they can make better decisions if they actually use data as a strategic asset. The best way to capitalize on this value is through a productized data platform. Data platforms not only optimize data management but also create the foundation for leveraging more advanced technologies such as artificial intelligence (AI). They also help with systematic and scalable options for data democratization giving end users the abilities to access, curate, and operationalize their data into insights.
Understanding Data Platforms
A data platform is a full-fledged system which connects, controls, and delivers data from various sources to users and applications. It has data ingestion, storage, processing, and analytics capabilities. In its product form, a data platform is built with user-centric principles to serve the needs of those who consume it - namely, data analysts, engineers, scientists, and AI algorithms, to name a few.
The Synergy Between Data Platforms and AI
AI runs on data. The platform works best when it has varied and high-quality, contextualized data to access. The following are the ways that a powerful data platform can elevate AI initiatives:
1. Data Accessibility and Integration
AI models need substantial resources not only from large amounts of data but also from a variety of data sources. A data platform combines data coming in from different systems and serves as a single, unified data organizer and aggregator. This integration of various data sources stands as the invariable element by which AI can, at any given time, effortlessly and seamlessly learn from all the extensive datasets. The algorithms are programmed to learn from these datasets, thereby enhancing their performance over time without any human intervention.
2. Data Quality and Consistency
The precision of AI models comes primarily from the quality and coherence of the data they are trained with. A data platform is responsible for controlling data quality standards so that the data is cleaned, consistent, and controlled. The quality of data being leveraged by AI models is essential to ensuring confidence and credibility in their results.
3. Scalability
As companies expand, the volume of their data also grows. A product-centric data platform is intrinsically scalable, thereby handling more loads of data without affecting its performance. AI needs real-time processing ability as it relies on big datasets that allow for building more complex and accurate models.
4. Near Real-Time Data Processing
There are many AI applications such as predictive maintenance or real-time analytics requiring up-to-date data. A data platform supports real-time data processing which ensures that the latest information is delivered to AI models for prompt and useful insights. This capability is vital for applications such as fraud detection and customer experience management delivered at the point of decision.
5. Data Security and Compliance
Data security and regulatory compliance are very important in every organization. A data platform contains fortified security measures and complies with data protection regulations. This security is essential for AI systems that deal with sensitive information such as healthcare or financial information.
6. Advanced Analytics
Today's data platform users need advanced capabilities such as cohort analysis, sentiment analysis, and time series analysis. These capabilities aid data scientists and analysts in researching the data to make a better feature selection. They also help them move forward with their next AI model development step and uplift the performance level of AI solutions.
The Productization of a Data Platform
When building a data platform as a product, it’s essential to adopt a product management mindset. Here are some of the key considerations:
1. User-Centric Design
Know what your end-users - data scientists, business analysts, or AI engineers- want. The platform should be user-friendly, intuitive, and must have all relevant features that users need.
2. Flexibility and Customization
The data landscape is continuously evolving. Make sure that your data platform is agile and can flex to process new sources that a new technology or business demands.
3. Robust Infrastructure
Build a powerful infrastructure that works with high availability, scalability, and performance. When it comes to creating scalable and cost-efficient data platforms, cloud-based solutions are almost always a great choice.
4. Marketing and User Adoption
Develop a marketing strategy to promote the platform and attract users. Create an effective onboarding process to help new users get started quickly and understand the platform’s capabilities. Evangelize its progress and wins to recruit and expand stakeholders in the business who will benefit from it.
5. Define Clear Objectives and Scope
Define the primary objectives of the platform. What business problems should it be solving? What value does it provide to users? What small wins could it achieve to demonstrate its value to leadership? Set measurable goals to track the platform’s success and guide its development. Avoid scope creep by regularly reviewing and adjusting priorities based on user feedback and market changes.
6. Building a Community
Foster a community around the platform to encourage user engagement. Recognize and reward user contributions to build a sense of community and shared ownership.
Conclusion
Treating a data platform as a product is like pulling out the multi-tool in your pocket for any new initiative involving AI. Organizations can fast-track their AI journey and unlock massive business value by delivering a scalable, secure, and customer-centric data infrastructure. Adopting this AI-first approach not only improves the quality of AI used, it also promotes development of a data-driven culture that prepares organizations for long-term success.
About Guidewire Data Platform
A unified, property and casualty (P&C)-centric data platform provided as a cloud core service for Guidewire Cloud Platform that enables access, curation, and insight into an insurer’s core system data.
Guidewire Data Platform unlocks the unlimited potential of data to deliver insights to insurers. It creates the most complete view of your Guidewire InsuranceSuite data, curates insights-ready data for analytics, and guides business outcomes with reporting, visualization, and prediction capabilities. Guidewire Data Platform captures and records business critical P&C data to be leveraged in analytics use cases, whether that's with the Guidewire analytics products or through access for AI/machine learning pipelines. Its curation capabilities allow for data and information technology leaders at P&C insurers to focus on delivering P&C insights rather than building data infrastructure.