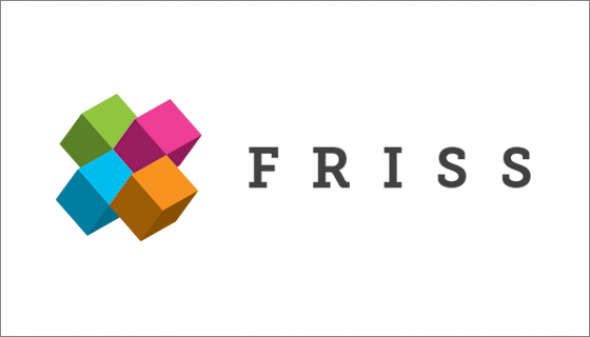
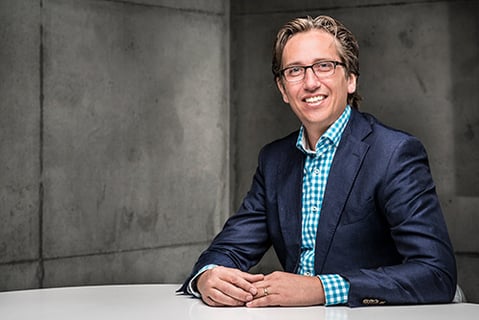
Insurance companies consume terabytes of information every day in the form of digital data. Valuable data is being provided by more and more sources, which quickly paint a reliable picture of a risk or a submitted claim and make it possible to filter out unwanted risks and fraudulent claims. State-of-the-art technology is required to process this influx of information and convert it into applicable knowledge and insights. This calls for intelligent software capable of processing information quickly, learning independently, drawing smart conclusions, and making recommendations – similar to a human, but smarter and more efficient. We are talking about artificial intelligence (AI).
Three types of AI
We can identify roughly three types of artificial intelligence. The first benefits everyone and is known as general AI. Examples of this type of AI include natural language processing, facial recognition, and augmented reality. In the latter, the physical world is overlaid with elements from the virtual world. We use elements of general AI to support specific process in the two other forms of AI.
The second form, product-based AI, involves specific products, such as systems designed to identify and deactivate computer viruses, programs that filter e-mail spam, and programs that detect fraud patterns. The latter is extremely valuable to us. The more we feed AI with information about fraud cases, the better it becomes at this specific task. The systems learn by doing and by drawing on feedback from many different users.
The third type of AI is custom or domain-specific AI. In short, AI that is trained for usage in a specific niche. An example from within the insurance sector is AI that has been taught to identify fraud patterns and is linked to Straight-Through Processing (STP) and the characteristics of a single insurance company: the specific products, target groups, distribution channels, and claims processes. This allows us to determine how AI can best be integrated into the business operations of an individual insurance company.
AI and image analysis
Combining all three types of AI often leads to the best results; for instance, using AI to analyse images in a STP environment while simultaneously checking for fraud. Insurance companies receive huge amounts of visual material, which is used to illustrate claims and support the claims process (e.g. determining the legitimacy of a claim). The photos are submitted by policyholders, intermediaries, repair shops, and experts, to illustrate damage (e.g. car damage, glass damage, or fire damage) or as evidence that lost or stolen items were indeed in the possession of the owner (e.g. jewellery, clothing, or cameras).
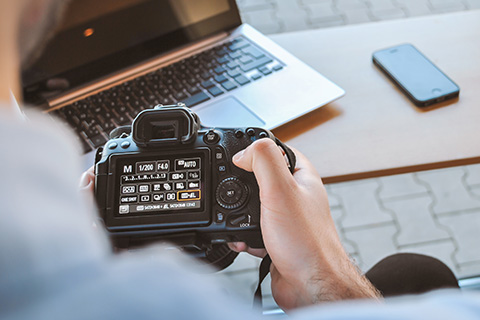
Claim assessment
In order to optimize the use of photographs, it must be determined that the right photos were submitted and that the damaged object was photographed from every angle. It is also important to determine that the object in the photo is indeed the insured object, that older damage is not being included in the claim, and that the photo has not been used before, downloaded from the internet, or photo-shopped. The next step is to determine the damage and the costs of repairing it. Is the car a total loss? How bad is the fire damage? Are there any visible indications of fraud?
These analyses can be made with the help of the three AI types listed above. General AI is trained with millions of images to identify general objects like cars, windows, buildings, etc. The huge quantity of data and the impressive computational power means these analyses are carried out at lightning speed and with great accuracy. Product-based AI is taught using specific claims (e.g. glass damage) to determine the amount of damage and to identify potential fraud. As a final step, AI can also be trained with various machine learning algorithms in the specific application domain: in this case, the process used by a specific insurance company.
Combining these three types of AI ensures smooth and efficient claims processing. The longer AI is used and the more data and feedback it receives about the claims process, the better and faster it will work. This will speed up the claims assessment process and may even lead to a fully automated process in some cases, such as STP. An added benefit is that fraudulent claims are filtered out more efficiently.
In addition to image analytics, there are dozens of AI types currently available and under development. Insurance companies can use these technologies to improve and streamline their risk analysis and fraud identification processes. In my next blog post I will elaborate on this subject, using some of our fraud prevention cases as an example.