
Insurance pricing can feel like a neverending headache. But why?
To start, it’s always an attempt to predict the future. When an insurance policy is sold, no one knows exactly how much it will cost in the end. Claims might be small, large, or none at all. To manage this uncertainty, insurance companies use risk pooling, where many customers pay premiums into a shared fund. While the cost of any one policy is unknown, pooling many policies together helps insurers cover costs and stay in business.
Even so, financial uncertainty is a known problem, one with much research and experience invested in solving it. In fact, there’s a whole science associated with managing it. But it’s not the only reason insurers find themselves investing significant resources into maintaining competitive prices while remaining unsatisfied or unsure of the results.
"Insurance pricing involves three distinct groups of professionals, each with different mandates and concerns."
Which raises the following question – what makes insurance pricing a difficult business problem to solve?
In reality, the biggest issue isn’t the science, but the process. Insurance pricing involves three distinct groups of professionals, each with different mandates and concerns. If sufficient attention isn’t paid to the intersections between these groups, then insurance pricing will remain slow, inefficient, and painful, if not also inaccurate.
This is what we explore in the first installment of our three-part series on insurance pricing challenges and how to solve them.
Insurance pricing stakeholders: How each plays a vital role in the pricing process
Insurance is a business, and in any company, there are business owners responsible for performance. These are the first stakeholders. They may hold different positions in different organizational structures – Line of Business Product Owner, Chief Underwriting Officer, Chief of Insurance Operations, etc. – but the fundamental role is the key. These people are responsible for the profit and loss of specific insurance lines of business.
Business owners manage the totality of insurance operations, from sales and distribution to marketing, to customer management, and to claims operations. To successfully manage the business, they’re also responsible for the premiums charged. Business owners must manage the inflows (premiums) and outflows (claims and expenses) such that a profitable business emerges.
As in any business endeavor, these people rely on others. In insurance pricing, the first challenge is having the right systems in place to run the business on a day-to-day basis. This means having a rating engine that can calculate premiums for given customers as needed.
The second group of stakeholders is IT professionals who build and maintain these products. They ensure that rating engines are efficient, reliable, and capable of delivering accurate rates.
But what rates should those be? Here, the business owners rely on actuaries and other analytics professionals to review the data and provide appropriate rates – ones that will protect the insurer’s solvency and competitiveness in a crowded market.
Analytics teams are the third set of stakeholders. They face the forward-looking nature of insurance pricing head-on, bringing data science to the challenge.
To understand how these groups collaborate in practice – and why disconnects arise between them – it helps to explore how insurance pricing functions are organized.
The structure of insurance pricing activities
The diagram below shows a high-level view of the pricing function. In particular, it highlights the ownership of different parts of the process, noting the different cadences of the activities.
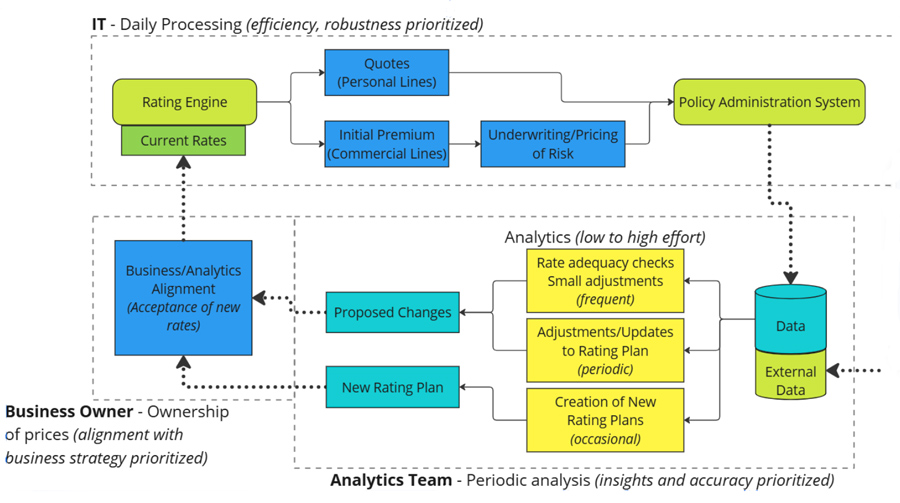
The top box represents the daily operations of insurance, where policies are quoted and sold to prospective customers. This is a critical function that takes the rates being charged (“current rates”) as a given. The diagram also notes the close connection between the rating engine and the policy administration system, both of which enable the insurer to manage day-to-day operations. These highly technical IT products must be built and maintained by IT professionals.
Where do the current rates that the rating engine uses come from? The lower-right box shows the activity of the Analytics team. Data is extracted from the operating systems, perhaps supplemented with external data, and analyzed in a variety of ways, depending on the purpose of the project.
Sometimes, on-going performance is monitored and tweaked for trends, such as inflation and medical costs. Other times, more significant reviews of rating plans are done. Occasionally, there’s a need to develop a new set of rates in advance, either because a new product is being offered or because the existing rating plan is just that old. In each case, historical data is extracted and analyzed – and changes to the rates are proposed.
Business owners rely heavily on IT professionals and analytics teams for the data and systems needed to do their jobs effectively. And yet, as the ones responsible for profit and loss, they define the goals and strategies in which the other teams operate. They review and challenge proposed changes to pricing to ensure alignment with strategy. And, if they value analytics, they stay informed of the detailed insights provided by the analytics teams.
Yet, despite the importance of their collaboration, these stakeholders often face communication barriers due to differences in terminology and viewpoints.
Navigating language gaps across pricing, data, and technology
Within their spheres of influence, IT professionals and analytics teams are highly qualified. Given proper direction from the business owners, they do their jobs well. The language problem arises because correct prices aren’t just determined and implemented. They’re maintained over time, analyzed and adjusted to remain optimal in changing economic and competitive conditions.
This means that each group must concern themselves not just with what’s within their outlined box, but also with the dotted-line connections between the boxes.
Efficient and robust operational systems can become pain points for analytics teams if they don’t easily provide data for analysis. In addition, analytics teams become less effective when they provide results that business owners can’t easily consume, review, and challenge. If business owners cannot efficiently and quickly change current rates, they’re hamstrung in keeping up with changing market conditions. And the issue isn’t simply siloed business functions with limited visions of their responsibilities.
The issue is that these three groups speak different languages.
Certainly, the “language” referred to here includes the professional jargon and concepts familiar to, and only to, the three groups. Thus, it’s critical to have curious professionals who care about communicating with other groups across professional lines.
- IT professionals who can translate business requirements into efficient systems and tools – and who take the data needed for modern analytics seriously.
- Analytics professionals who can program enough to assist with the extraction of data and the creation of required pipelines. Also, those who have a passion for seeing their output understood and benefiting the business.
- Business owners who have a clear understanding of their own strategy and who can communicate it accordingly, translating it into terms that other groups can act on.
But the language problem goes deeper in two specific ways.
Modern analytics rely on complicated system architectures, the data required, and the analytical tools used to interpret that data. The same could be said for rating engines and policy administration systems. However, these system architectures aren’t the same. They’re often literally written in different programming languages.
In addition, data structures, which allow fast daily processing and efficient storage, are radically different from the tabular data structures needed for analytics.
"Modern analytics rely on complicated system architectures, the data required, and the analytical tools used to interpret that data."
When groups optimize solely for the priorities of their own responsibilities, disconnects result. And the language differences mean that the disconnects aren’t trivial. Referring back to the diagram, reasonably well-developed tools and processes exist within each dotted-box. What makes insurance pricing difficult are the dotted arrows between the boxes.
To add to this challenge, good insurance pricing is about maintaining those prices over time as the economy changes and competitors evolve. Consequently, analytics teams constantly want new data to produce new insights. Business owners frequently engage analytics to review their options and decide what change to implement, as well as what course to take. And so, the “current rates” in a rating engine are constantly subject to change, making the need for clear communication even more important.
But how do you actually get everyone on the same page?
From silos to synergy: How to align teams for pricing success
First and foremost, an appreciation of the disconnects in your own pricing function is needed. Focus on the transitions.
- Do you have systems and tools for extracting data into formats that analytics teams can use? Build pipelines for repeated processes.
- Do you have an environment where business owners and analytics teams can review proposed changes, examine the impact to existing business, ask questions about the effect of possible changes, etc.?
- Preserving controls over what’s in production, can business owners and their teams change and manage rates directly, without needing to kick-off an IT project?
To the extent possible, look for platforms that span these capabilities, as these have likely already been built to facilitate the transitions. Consider the second bullet point above as an example. What if the place where changes can be reviewed is on the same architecture as the rating engine, so that approved changes can be implemented with the touch of a button? What if it was also aligned with the analytical tools, so it could import the output of predictive models directly? What if it was integrated with multiple data structures, so that importing in-force business is a given, rather than an extra project?
There’s no technological barrier to gaining these kinds of efficiencies. What has been lacking is an appreciation of the size of the problem and a realization that it can be solved. There are difficulties – the different data structures, system architectures, and professional expertise are real – but the difficult problems tend to be the ones worth solving. Since good insurance pricing is about maintaining those prices, each improvement compounds in value over time. There’s no reason that, in today’s world, a more efficient pricing process can’t be achieved.
Stay tuned for part two – deeper insights on insurance pricing challenges and how to overcome them.